How to Detect Deepfakes: Expert Tips and Tools
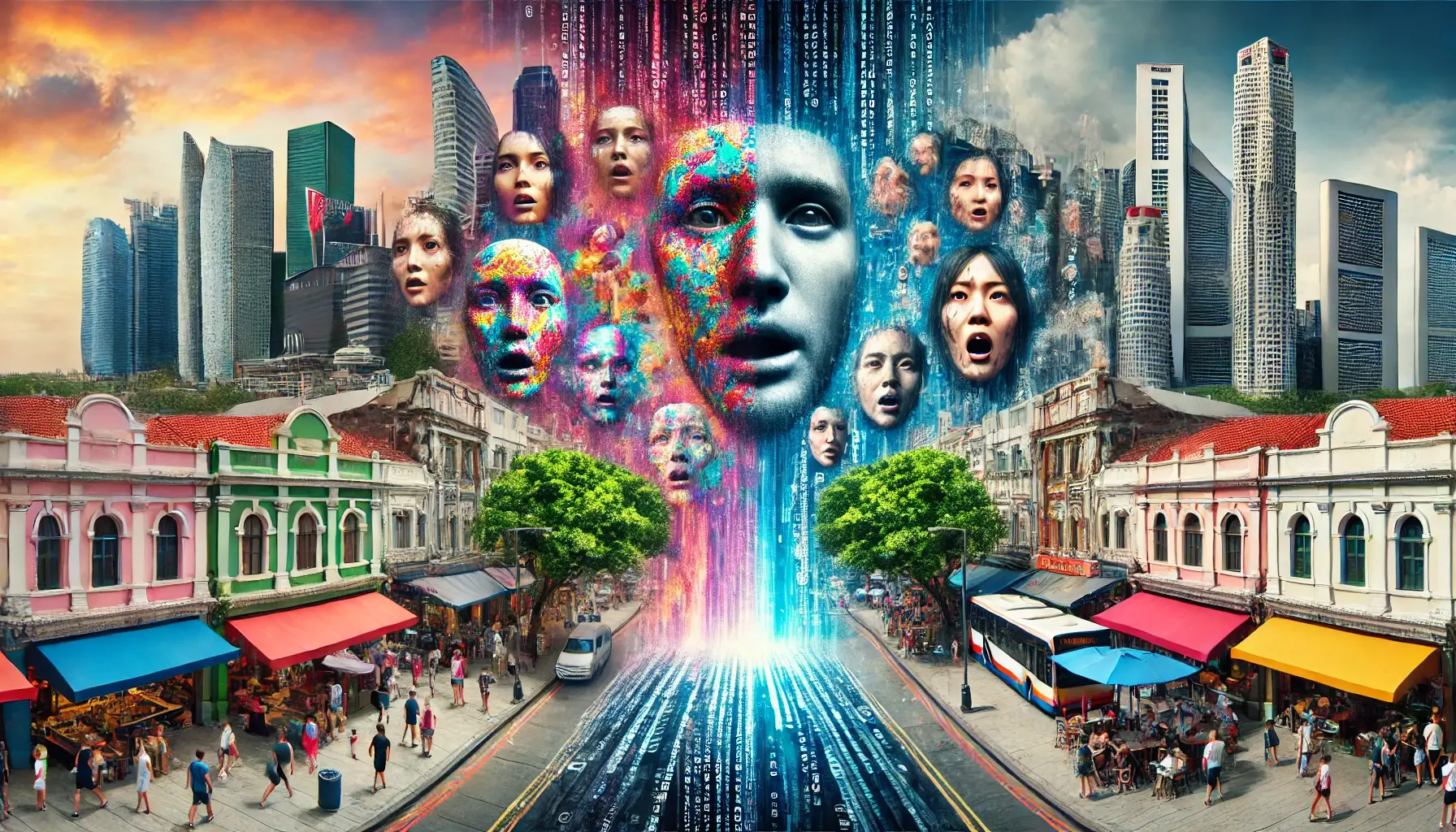
Turn any text into audio
Peech is a text-to-speech tool that quickly converts PDFs, eBooks, articles, and more into high-quality audio
Try It Now
Detecting deepfake videos, which are AI-generated manipulations of images, videos, or audio, is becoming increasingly essential in our media-driven society. To recognize deepfakes, look for subtle inconsistencies such as unnatural facial movements, odd lighting effects, or mismatched voice and lip movements. These signs indicate the presence of algorithmic alterations that may not be immediately apparent.
Using tools designed to detect phoneme and viseme mismatches can also help identify deepfakes. These AI tools exploit the inconsistencies between mouth movements (visemes) and spoken sounds (phonemes) to detect manipulations. Additionally, enhancing your media literacy skills by zooming out and examining broader contexts rather than focusing obsessively on minute details can be a powerful strategy.
Understanding deepfakes’ potential to distort reality is crucial in an age where misinformation spreads rapidly. By employing a combination of visual analysis and advanced AI detection tools, individuals can better safeguard themselves from deceptive media.
Understanding Deepfakes
Deepfakes are AI-generated content that can manipulate the appearance, voice, or actions of individuals in videos and images. Deepfake generation involves advanced technologies like Generative Adversarial Networks (GANs) to create or manipulate media, posing challenges for detection due to their evolving nature. This section provides a detailed look at the technology behind deepfakes, their societal implications, and the ongoing efforts to detect them.
Evolution of Deepfake Technology
Deepfakes utilize AI and machine learning, particularly generative adversarial networks (GANs), to create realistic fake videos and images. Initially, they required extensive computing resources and technical skills, but advancements have made the tools more accessible.
The latest progress in this field includes more user-friendly software and improved algorithms. This has made deepfakes more convincing and harder to detect. However, advancements in deepfake detection models have also been significant, addressing challenges such as dataset diversity and the risk of discrimination. Early deepfakes exhibited noticeable artifacts, such as unnatural eye movements or inconsistent lighting, but newer iterations have minimized these flaws.
Implications of Deepfakes in Society
The rise of deepfakes poses significant challenges for privacy, cybersecurity, and fake news. They can be used to create misleading content that appears authentic, complicating the efforts of fact-checkers and tarnishing reputations.
Privacy concerns are paramount; individuals may find their likeness used inappropriately, leading to potential emotional and social harm. In cybersecurity, deepfakes can be weaponized, presenting risks of identity theft and fraud.
Their use in misinformation campaigns can destabilize trust in media and institutions. Solutions include better detection systems and regulatory frameworks to mitigate these risks.
Deepfake Detection Challenge (DFDC)
The Deepfake Detection Challenge (DFDC), organized by major tech companies like AWS and Microsoft, aimed to enhance methods for identifying deepfakes. This research experiment provided a benchmark dataset for developing new detection algorithms.
Participants in the challenge used various machine learning techniques to improve the accuracy of deepfake recognition. The challenge underscored the difficulty of distinguishing AI-generated content from genuine media, highlighting the need for ongoing innovation.
Continuous research and collaboration between organizations are vital for staying ahead in the fight against deepfake proliferation and enhancing the robustness of detection tools.
Techniques for Detecting Deepfakes
Detecting deepfakes involves analyzing various elements such as visual clues, audio inconsistencies, and metadata. There are several detection tools that utilize machine learning and deep learning to analyze large datasets of altered media, aiming to identify unnatural patterns that indicate artificial creation. Utilizing artificial intelligence and human oversight can significantly enhance the accuracy and reliability of detection methods.
Visual and Audio Analysis
Visual and audio analysis focuses on identifying anomalies that are difficult to replicate in AI-generated images and videos. Specific visual indicators include unusual blinking patterns, inconsistent lighting, and unnatural facial expressions. Analyzing the eyes can be particularly revealing, as AI often struggles with accurate blink rates and eye movements.
In audio analysis, detection methods look at synchronization between lips and speech, tone consistency, and background noise discrepancies. Identifying these manipulation clues helps to distinguish real videos from altered ones.
Artificial Intelligence in Detection
AI and deep learning technologies play a crucial role in deepfake detection. Machine learning algorithms can be trained on vast datasets of real and fake content to improve their ability to identify inconsistencies. These algorithms compare various factors such as facial geometry, movement patterns, and texture analysis to detect deepfake manipulation.
Tools like Sentinel use sophisticated AI models to protect enterprises and organizations by identifying manipulated videos and images. AI-powered solutions continually evolve, ensuring that detection methods stay ahead of deepfake creation techniques.
Beyond the Algorithm: Human Oversight
Human oversight remains an essential component in the battle against deepfakes. While algorithms can detect many clues and inconsistencies, human expertise is invaluable in flagging subtle and context-specific anomalies. Experts analyze metadata, such as timestamps and encoding information, to identify possible manipulation.
Incorporating a combination of automated systems and human scrutiny offers a more robust defense. Human oversight helps validate the findings of AI systems, ensuring higher accuracy in distinguishing real videos from deepfakes. This collaborative approach is critical in industries such as media, defense, and enterprise IT.